Artificial Intelligence (AI) algorithms have become an integral part of our daily lives, influencing decisions ranging from what news articles we see to who gets approved for loans. However, these algorithms are not immune to bias, often reflecting the prejudices present in the data they are trained on. Navigating bias in AI algorithms is crucial to ensure fair and equitable outcomes for everyone. In this article, we delve into the complexities of bias in AI and explore strategies to mitigate its effects.
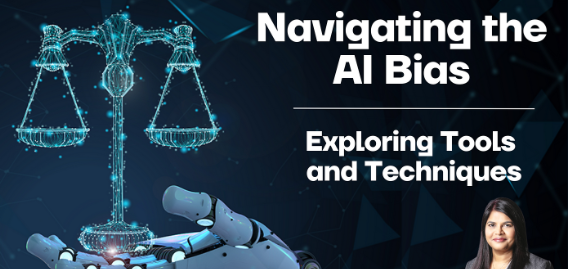
Understanding Bias in AI Algorithms
Bias in AI algorithms can manifest in various forms, including but not limited to:
Data Bias:
When training data is unrepresentative or skewed, leading the algorithm to make inaccurate or unfair predictions.
Algorithmic Bias:
Occurs when the design or implementation of the algorithm itself introduces unfairness or discrimination.
Implicit Bias:
Reflects the unconscious biases of the individuals involved in designing, training, and deploying AI systems.
Impact of Bias
The consequences of bias in AI algorithms can be far-reaching, affecting individuals and communities in profound ways:
- Social Inequities: Biased algorithms can perpetuate and exacerbate existing social inequalities, such as racial discrimination in hiring or sentencing.
- Lack of Diversity: Bias can lead to underrepresentation or misrepresentation of certain groups, reinforcing stereotypes and limiting opportunities for marginalized communities.
Strategies to Mitigate Bias
Addressing bias in AI algorithms requires a multi-faceted approach, encompassing various stages of the AI development lifecycle:

Diverse and Representative Data:
Ensure that training data is comprehensive and reflects the diversity of the population it seeks to serve.
Algorithm Transparency:
Make algorithms transparent and explainable, allowing stakeholders to understand how decisions are made and identify potential biases.
Bias Detection and Mitigation:
Implement mechanisms to detect and mitigate bias throughout the AI system’s lifecycle, from data collection to model deployment.
Ethical Oversight:
Establish ethical guidelines and frameworks for the development and deployment of AI systems, emphasizing principles of fairness, accountability, and transparency.
FAQs
1. How do biases enter AI algorithms?
Biases can enter AI algorithms through the data they are trained on, the design of the algorithms themselves, and the implicit biases of individuals involved in the development process.
2. Can bias in AI algorithms be eliminated?
While the complete elimination of bias may be challenging, it can be mitigated through careful attention to data collection, algorithm design, and ethical considerations throughout the AI development lifecycle.
3. What are the potential consequences of biased AI algorithms?
Biased AI algorithms can perpetuate social inequities, reinforce stereotypes, and result in unfair treatment of individuals or groups, leading to mistrust and negative impacts on society.
4. How can individuals advocate for fairer AI algorithms?
Individuals can advocate for fairer AI algorithms by raising awareness about bias, supporting diversity in AI research and development, and holding organizations accountable for ethical AI practices.
Conclusion
Navigating bias in AI algorithms is a complex but essential endeavor. By understanding the sources and impacts of bias and implementing strategies to mitigate its effects, we can strive towards creating AI systems that are fair, inclusive, and beneficial for all. As we continue to advance AI technology, it is imperative to prioritize ethical considerations and ensure that AI serves the best interests of humanity.